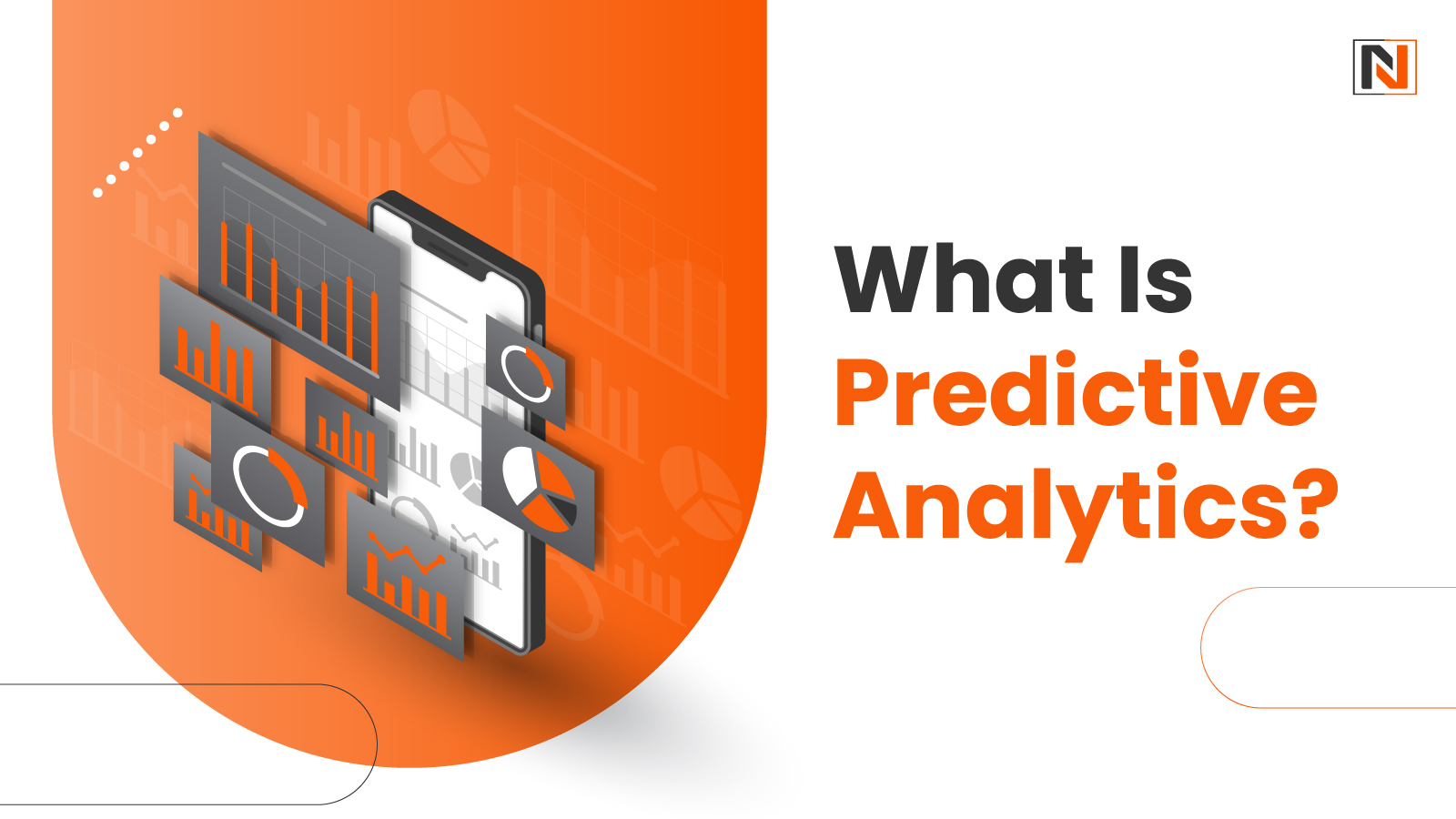
Predictive analytics leverages statistical and modeling techniques to anticipate future outcomes by analyzing both current and historical data patterns. These patterns are studied and projected to assess the probability of their recurrence.
Businesses are empowered by predictive analytics to optimize operations and evaluate the potential of new product investments. Investors rely on it to identify promising opportunities.
Online retailers use predictive analytics to personalize purchase recommendations for users to increase sales and enhance customer experience.
Why is Predictive Analytics Important?
Organizations increasingly leverage predictive analytics to tackle complex challenges and uncover fresh opportunities. Key applications include:
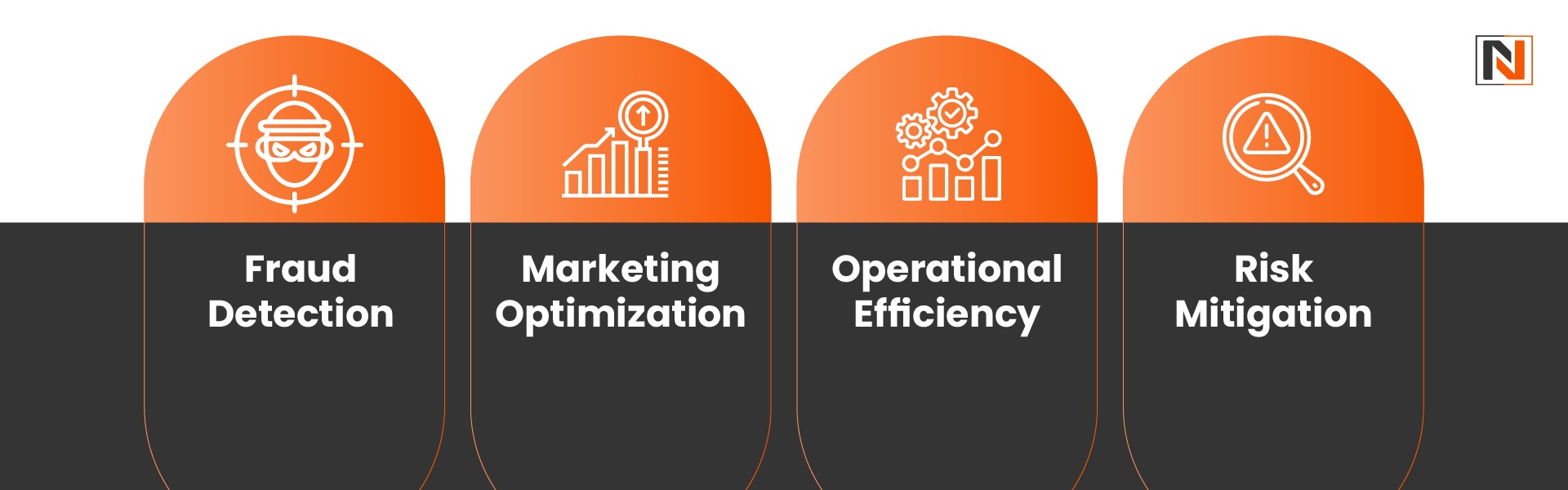
- Fraud Detection
By integrating various analytical methods, predictive analytics enhances the ability to identify patterns, detect criminal activities, and prevent fraudulent actions. High-performance behavioral analytics monitors network activity in real-time as cybersecurity concerns rise, identifying anomalies that could signal fraud, zero-day vulnerabilities, or advanced persistent threats.
- Marketing Optimization
Predictive analytics helps forecast customer responses, purchases, and cross-sell opportunities. Businesses utilize predictive models to attract, retain, and expand their most valuable customer base, which enables them to create more effective marketing campaigns.
- Operational Efficiency
Predictive models assist companies in forecasting inventory and managing resources. Airlines rely on predictive analytics to determine ticket pricing, while hotels use it to estimate nightly guest numbers, maximizing occupancy and revenue. This empowers organizations to streamline operations and boost efficiency.
- Risk Mitigation
Credit scores, a prominent example of predictive analytics, assess the likelihood of default based on a buyer’s creditworthiness. These scores, derived from predictive models, are widely used in financial transactions. Additional applications include managing insurance claims and collections helping organizations mitigate risks effectively.
Did You Know?
According to Markets and Markets, The global Predictive Analytics Market, valued at $10.5 billion in 2021, is expected to grow to $28.1 billion by 2026.
According to Statista, 92% of healthcare leaders in Singapore have either already adopted or are in the process of adopting predictive analytics in their organizations, marking the highest adoption rate among all countries surveyed.
How Does Predictive Analytics Work?
Data scientists, experts in data analysis and modeling, play a crucial role in predictive analytics. They use predictive models to uncover relationships between various elements in chosen datasets. After data collection, a statistical model is created, trained, and refined to generate predictions. The expertise and experience of data scientists are essential in ensuring the accuracy and reliability of the predictions.
The process for building predictive analytics frameworks typically involves five key steps:
1. Define the Problem: Every prediction begins with a clear thesis and a set of requirements. For example, can a predictive analytics model detect fraud? Optimize inventory for the holiday season? Forecast potential flood levels due to severe weather? Clearly defining the problem helps determine which predictive analytics method to use.
2. Acquire and Organize Data: Organizations may have vast amounts of historical data or continuous data streams from customer interactions. Before developing predictive models, identifying the data flow and organizing the datasets in a repository, such as a data warehouse like BigQuery, is essential.
3. Pre-process Data: Raw data is not immediately useful in its raw form. To prepare it for predictive modeling, it must be cleaned, anomalies removed, missing data filled in, and outliers caused by input or measurement errors eliminated.
4. Develop Predictive Models: Depending on the problem and the dataset, data scientists use various tools and techniques to build predictive models. Common methods include machine learning, regression models, and decision trees.
5. Validate and Deploy Results: After developing a model, its accuracy must be tested and refined. Once the model delivers satisfactory results, it’s deployed for stakeholders to access, often through apps, websites, or data dashboards.
Must Read – Why Cybersecurity Should Be a Top Priority for Your Business
Types of Predictive Modeling
Predictive analytics models are designed to assess historical data, discover patterns, observe trends, and use that information to predict future trends. Popular predictive analytics models include classification, clustering, and time series models.
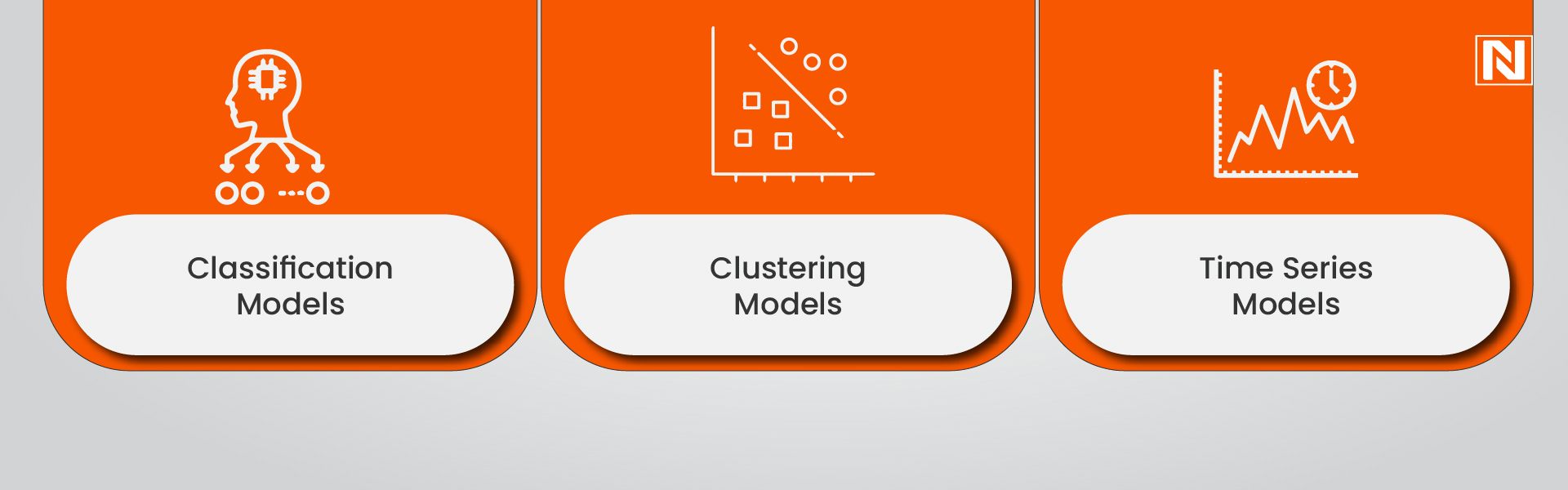
- Classification Models
Classification models are part of supervised machine learning. These models categorize data by analyzing patterns from historical datasets and uncovering relationships within the data. For instance, they can segment customers or prospects into distinct groups for targeted marketing.
Additionally, they excel at answering binary questions, such as “yes or no” or “true or false.” Fraud detection and credit risk evaluation are common applications. Examples of classification models include logistic regression, decision trees, random forest, neural networks, and Naïve Bayes.
- Clustering Models
Clustering models are a part of unsupervised learning. They group data based on shared characteristics, making them useful for identifying similarities. For example, an e-commerce platform can use clustering to segment customers into groups with similar traits, enabling tailored marketing strategies for each group.
Widely used clustering algorithms include k-means clustering, mean-shift clustering, DBSCAN (density-based spatial clustering of applications with noise), expectation-maximization (EM) clustering with Gaussian Mixture Models (GMM), and hierarchical clustering.
- Time Series Models
Time series models analyze data collected over specific time intervals, such as daily, weekly, or monthly, to identify patterns like seasonality, trends, and cycles. These insights often guide the selection of transformations or appropriate model types.
Commonly used models include autoregressive (AR), moving average (MA), ARMA, and ARIMA. For instance, a call center might use a time series model to predict call volumes by hour, helping optimize staffing and operations.
Must Read – How to Choose the Right Cloud Service for Your Business Needs
Predictive Analytics Industry Use Cases
Predictive analytics can be applied across various industries to address various business challenges. Some industry-specific use cases demonstrate how predictive analytics can drive informed decision-making in real-world scenarios.
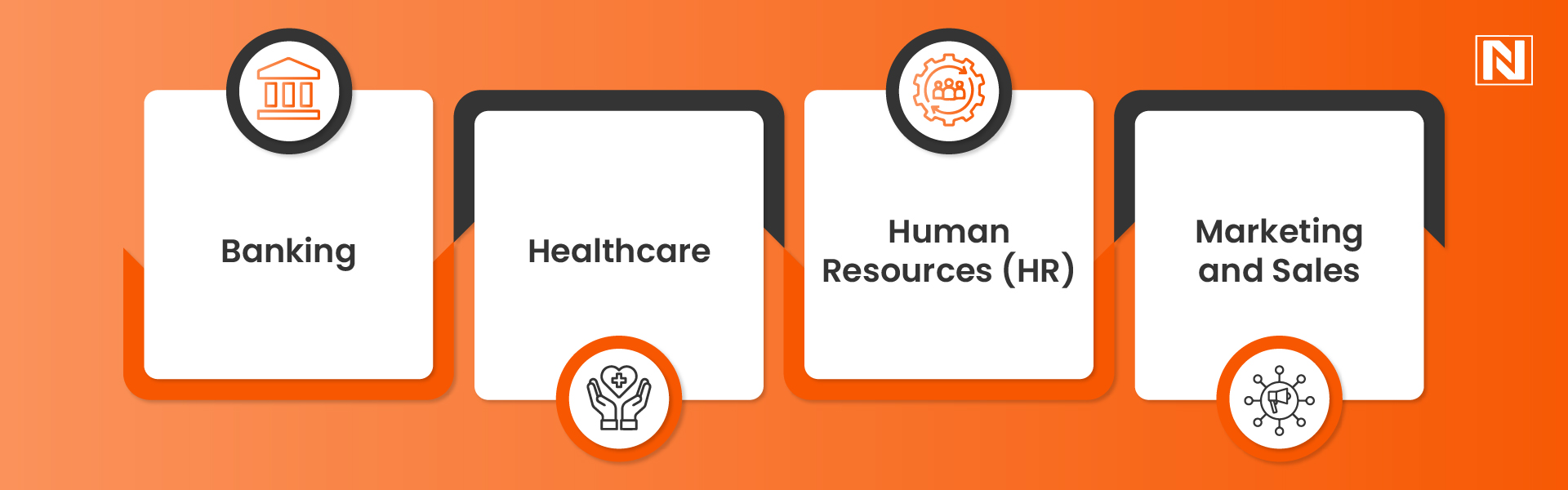
1. Banking: Financial institutions leverage machine learning and quantitative tools to predict customer behaviors and trends. This helps banks answer critical questions such as who is likely to default on a loan, which customers present high or low risks, which are the most profitable to target with resources and marketing, and which transactions might be fraudulent.
2. Healthcare: In healthcare, predictive analytics plays a vital role in identifying and managing chronic illnesses and tracking specific conditions like sepsis. Geisinger Health used predictive models on health records from over 10,000 sepsis patients to improve diagnosis and treatment. This model successfully predicted patients with a high survival rate, showcasing the power of data-driven care management.
3. Human Resources (HR): HR teams utilize predictive analytics and employee survey data to match the right candidates to positions, minimize turnover, and boost engagement. By combining quantitative and qualitative insights, businesses can lower recruiting costs and improve employee satisfaction, especially in unpredictable labor markets.
4. Marketing and Sales: While business intelligence reports offer insights into past sales performance, predictive analytics allows marketing and sales teams to engage proactively with clients throughout the customer journey.
For instance, churn prediction helps sales teams identify dissatisfied clients early, enabling retention efforts. Predictive data analysis also supports cross-selling strategies, such as personalized recommendations on e-commerce websites.
Wrap Up
Predictive analytics empowers businesses across various industries to make informed, data-driven decisions by forecasting future outcomes. By leveraging historical data and advanced modeling techniques, companies can enhance efficiency, reduce risks, and uncover new opportunities.
Contact Nirvana Lab for consultation and learn how predictive analytics can benefit your business.
Frequently Asked Questions
What is predictive analytics?
Predictive analytics uses statistical techniques and modeling to analyze current and historical data to forecast future outcomes, helping businesses make data-driven decisions and optimize performance.
How does predictive analytics benefit businesses?
Businesses use predictive analytics to enhance operational efficiency, detect fraud, personalize marketing, and manage risks. This allows them to make proactive decisions and improve overall performance.
What are the key types of predictive modeling?
The key types of predictive modeling are classification, clustering, and time series models. Classification models predict categorical outcomes, clustering models group similar data, and time series models analyze data over time to identify trends.
How do businesses use predictive analytics in marketing?
Businesses use predictive analytics to forecast customer behavior, personalize marketing strategies, optimize sales efforts, and identify opportunities for cross-selling.
What industries benefit from predictive analytics?
Predictive analytics is used across industries like banking, healthcare, human resources, and marketing to improve decision-making, reduce risks, and optimize operations, offering insights into customer behaviors, health trends, and employee management.